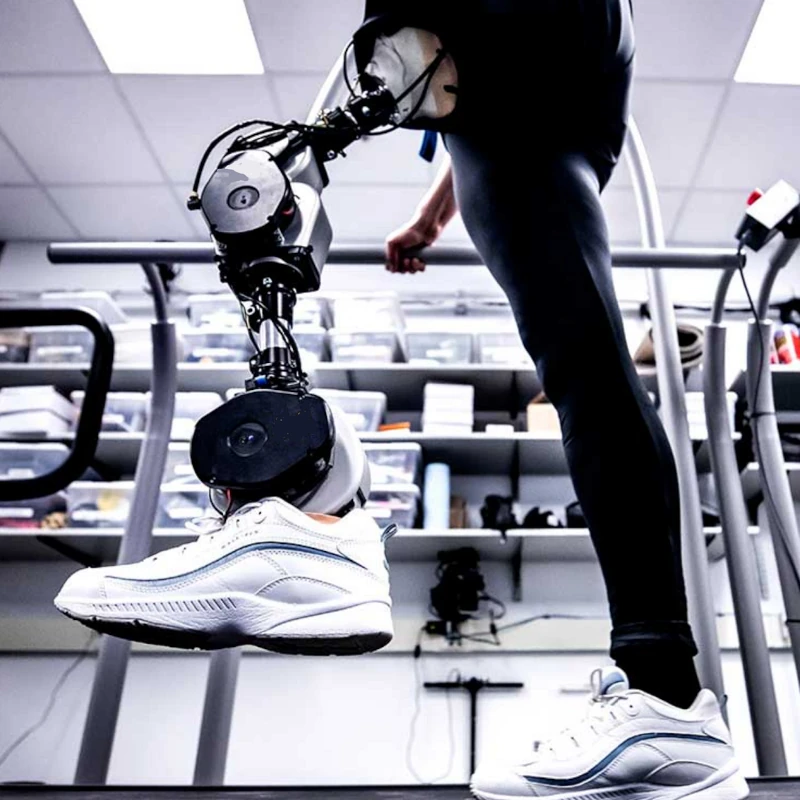
Osseointegration (OI) has been hailed as a “game-changer” in prosthetic technology. So has targeted muscle reinnervation (TMR), which supports more intuitive control of bionic devices. And the label also applies to fully powered limbs such as the Utah Bionic Leg, which use motors to add “muscle” to the prosthesis.
All three of these innovations will come together in a multi-year research project that’s just getting underway at the Shirley Ryan AbilityLab. Backed by $1.5 million from the Department of Defense, the supercharged initiative integrates boundary-pushing approaches in surgical technique, prosthesis design, and neuromuscular (EMG) signal-processing. Combining all three, says lead researcher Levi Hargrove, could pave the way for powered prosthetic legs that are lighter, more comfortable, and easier to use than anything currently available.
We had a chance to discuss the project with Hargrove, who helped pioneer similar systems for upper-limb prosthetic devices. Our conversation has been edited for clarity and length. Look for more details in the July issue of Amplitude.
What’s the new ground that’s being broken in this particular study?
We’ve been studying powered legs at the Shirley Ryan AbilityLab for about a decade now. These are motorized knee and ankle prostheses, primarily for individuals with above-knee amputation, and we’re looking at how they can use powered knees and ankles to restore better mobility—especially for activities like walking with a reciprocal gait, walking up and down stairs or up and down slopes, sit-to-stand transfers, etc. We’ve been doing that for a long time, and we have developed some prototypes.
Powered legs are generally a little bit heavier than non-powered devices, so we’re interested in using osseointegration for better suspension. We think the legs are going to feel lighter. That’s one component of it. And then the other component of the study is to try to figure out how we can send muscle signals back into the controller for these legs. We’ve been using neural signal decoding, or EMG decoding, and it’s been a real challenge to record lower-limb EMG signals inside of a socket. We’ve been doing this for decades with arms, but in legs, putting EMG sensors inside the socket and making them comfortable is very challenging. Individuals who have had osseointegration don’t need a socket, so this allows us to experiment with different ways to collect EMG signals in a more comfortable way and, hopefully, get a cleaner signal. And we hope this will allow us to make even better control algorithms for these legs.
And TMR will be the method of gathering the EMG signals, correct?
You are correct. TMR is the portal through which will have access to the information from the ankle muscles. Right. So, your gastroc muscle and your tibialis muscle, those are the muscles that allow you to lift your foot up and move your ankle up and down, dorsiflexion and plantar flexion. So we will use TMR to restore that EMG information onto the hamstrings. We’ll have the quadriceps that are natively innervated, or remaining after the amputation, and then we’ll use reinnervation for EMG signals to the gastroc and tibialis.
There’s a certain population of above-knee amputees out there who already are walking around with osseointegrated legs. What’s the added benefit of combining that with TMR?
While there are many people walking around with osseointegration, none of them are walking around with powered legs. They’re all using microprocessor passive legs, but none of them are using powered legs. And if you’re using a powered leg, you have to have better control. We’re using TMR to gather EMG information and send it to the powered legs. And our hypothesis is that, by not having a socket, we’re going to be able to collect much higher-quality EMG signals from the muscle, and this should provide significant clinical benefits when using the powered legs. Step-over-step stair climbing is one example. Or using a powered ankle to provide push-off while you’re walking quickly or upslope. Sit-to-stand transfers as well. So it’s the combination of the power leg with the really clean EMG control signals that we think will be game-changing.
What power leg will you be using?
We’ve been developing a powered-leg system at the Shirley Ryan AbilityLab as part of a grant sponsored by the National Institute on Disability, Independent Living, and Rehabilitation Research. This is a new hybrid leg that can operate in either power or passive mode. We designed it all from scratch, and we’ve been working with it for around eight years now, so we can interact with it very well. It’s a powered-knee, powered-ankle prosthesis. That will be the leg we’ll do most of our testing with.
How does the data collection occur? Will it all be in a lab setting, or will there be any sort of monitoring while people are going about their daily business? Tell me a little bit about what you’ll be measuring and how.
The combination of osseointegration and powered legs is not one of the indications for use from the FDA perspective, so we’ll have to do that in the lab only. After the individuals have the OI surgery, they’ll be set up with a state-of-the-art commercial prosthesis for daily use—a microprocessor knee, presumably. We’ll be doing all of the testing with the power leg in the lab.
As you know, rehabilitation for that procedure takes a while. It takes a few months for the implant to integrate, and then you have to progressively load to build up the integrity of the leg. After they’ve been fully osseointegrated, they’ll work with us for probably around six months. And they’ll come in a couple of times a month, usually for a day or half-day, and we’ll do experiments with a progressive level of difficulty. First we’ll fit them with the power device and train them how to walk with it. Then we’ll start collecting the EMG signals. And then we’ll start using AI to decode the EMG signals and all of the other sensors from the leg to make these these kinds of AI based controllers that are specifically trained for the user. And then they’ll come back in a few times to evaluate how well those models work for them.
We’ll also get more qualitative feedback on questions such as: How heavy does the device feel? How well do they like it in comparison to their everyday device? And in addition, we’ll use some of the standard outcome measures that are often used in lower limb prosthetics.
Are all eight of the test subjects people who are currently in a conventional socket prosthesis? Will they first need to get the OI procedure and then proceed to the testing phase? Or are there any who’ve already had OI and are just ready to get started?
We have funding to do four surgeries, and we have approval from our Institutional Review Board to evaluate individuals who have already had OI surgery. So it’ll be some combination of both.
Once this initial set of data comes in, and you’re able to analyze it,what are some potential next steps?
This is a necessary piece of work that’s being done to support the use of power legs. Fully powered leg systems, in my opinion, are going to become increasingly popular. It may not be this year, it may not be next year, but they’re coming, and this is part of the body of evidence that supports their use. If there are no safety events—and we don’t anticipate any—and if the higher-quality EMG signals provide the benefits we think they can, then this is going to be a trailblazing type of study.
If we project ahead to a commercial application: There’s the bionic leg itself, with the powered knee and powered ankle; there’s an EMG sensing system that’s collecting signals via TMR; and there’s an algorithm that is analyzing and translating those EMG signals. Would all of those be a fully integrated product? Or could there be any kind of modularity where you might be able to, say, apply the sensing unit to a different powered leg?
I think it could be modular. If we use upper-limb devices as an analogy, for example, the Coapt pattern recognition system is a modular EMG signal decoder that works with a number of different arms. It’s the same type of approach, where you’re using machine learning to decode EMG signals and other information that can be easily collected. I think you can provide a modular output, which basically is an instruction of the user’s intent to the prosthesis, that could be read by any prosthesis with its own controller, its own safety mechanisms, and so on. So it could be a modular system.
Alternatively, it could be built in as an entire, integrated system. But I think it would be more impactful if the EMG decoding aspect could be modular and applied to many devices in some sort of standardized way. That would be our hope and our preference.
In that scenario, suppose I have TMR surgery but I’m using a normal socket instead of OI. Could I use the same AI module to control a powered leg? Could that work?
Conceivably it could, if you could overcome the practical issues associated with measuring EMG signals in a socket. We would try to design control systems and an algorithmic approach that would work if you could overcome the sensing issues and come up with technology that allows those signals to be read in a relatively clean and comfortable way.
But I will also say that the weight of a power leg is always going to be a little bit more than a passive leg, just because there are motors and batteries in there. And the suspension you get from osseointegration is going to make these legs feel lighter—the perceived weight. So even if you get the benefit from the control system and the TMR and the neurosignal decoding in a socket, you’re going to get some additional benefit from the improved suspension provided by the OI.
At some point down the line, do you anticipate a below-knee application for this system? If it’s successful, and it’s modular, could you use the same EMG sensing and decoding technology in below-knee devices?
I think you could. I also think this approach could work with the AMI surgery that our colleagues are doing at MIT, Hugh Herr’s group and Matthew Carty. I don’t think it replaces that work, but I think it maybe could enhance it or help extract information from that. But yes, this conceivably could work in below-knee devices as well.